Artificial intelligence (AI) is creating massive waves across various industries, leading to unprecedented data generation and the need for innovative data storage solutions. As AI models become more complex, the demands on data storage systems escalate, challenging existing infrastructure to scale and adapt. The future of AI data storage is a fascinating landscape, featuring cutting-edge technologies and approaches set to redefine how data is stored, accessed, and managed. This text delves into prominent trends and innovations that are shaping the future of data storage for AI. Keep reading to uncover the significant strides being made in this vital sector.
At the same time, AI music is transforming the way we create and experience sound, making it easier than ever to generate unique, high-quality music for various projects.
The Rise of Quantum Computing and Its Impact on Data Storage
Quantum computing is revolutionizing data processing, enabling the solving of complex problems that classical computers cannot. This also presents new challenges and opportunities for data storage. Quantum-resistant encryption is being developed to protect data against quantum machines’ cracking capabilities, ensuring data security in this era.
Quantum computing is also influencing new data storage architectures that integrate quantum-processed data with classical systems. Despite its limitations, such as instability and high error rates, researchers are working to create stable qubits that maintain coherence for extended periods. As these technologies mature, they are expected to strengthen AI, predictive analytics, and big data harnessing, making quantum computing a driving force behind next-generation data storage solutions.
AI and Edge Computing: A Shift Towards Decentralized Data Solutions
The rise of IoT devices has driven the adoption of edge computing, where data is processed closer to its source. This reduces latency, making real-time AI applications like autonomous vehicles and predictive maintenance more efficient. By handling data locally, edge computing also eases bandwidth strain and enhances system resilience, allowing AI to function even during network disruptions.
Despite its benefits, edge computing presents challenges in data management and security. Devices must have sufficient processing power and storage, while decentralized networks require strict governance. To optimize AI at the edge, storage solutions for AI are being developed to ensure fast, secure, and efficient data access for real-time decision-making.
Breakthroughs in DNA Data Storage and Its Role in AI Data Handling
DNA data storage, a revolutionary concept in data storage, has the potential to store exabytes of data in a single gram of DNA. The integration of DNA data storage with AI capabilities could be transformative, as AI’s appetite for data aligns with DNA storage’s dense capacity.
DNA data storage is still in its infancy due to the high costs associated with synthesizing and sequencing DNA. Ongoing research is yielding more efficient methods of reading and writing genetic data, suggesting that DNA could soon become a viable storage medium for AI and other data-intensive applications. DNA data storage also offers sustainability, requiring less energy to maintain and a limitless shelf life.
Enhancing AI Data Security: Advanced Encryption and Blockchain Integration
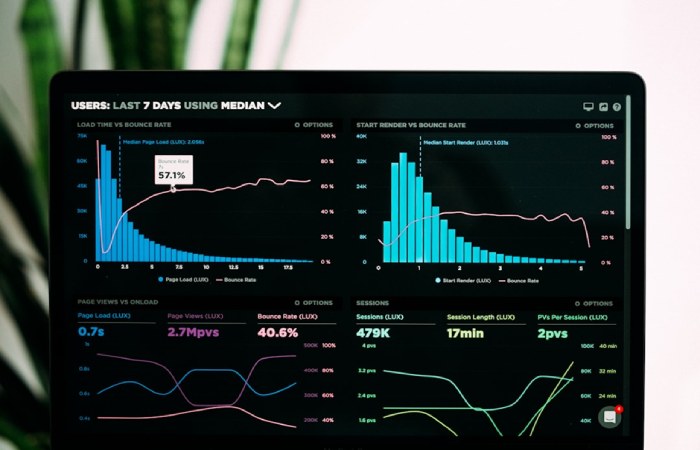
AI systems are transforming sectors like healthcare, finance, and national security, necessitating increased data security. Advanced encryption techniques, such as homomorphic encryption, are being developed to protect AI data, ensuring its security even during analysis. Blockchain technology is also being integrated into AI data storage to enhance trust and verifiability.
The dynamic relationship between AI and encryption requires balancing robust security with data accessibility by AI algorithms. Implementing these measures is crucial for fostering user trust in AI and ensuring the integrity and confidentiality of underlying data, a critical step towards sustainable and ethical AI growth.
Sustainable AI Data Centers: Green Computing Innovations and Practices
AI data centers are facing challenges in sustainability due to their high power consumption. Green computing initiatives aim to reduce their carbon footprint by using renewable energy sources and reducing reliance on fossil fuels. Cooling technology innovations, such as liquid immersion and geothermal cooling, are also crucial for creating sustainable AI data centers. Machine learning algorithms can optimize resource use and reduce wastage by adjusting workloads to meet actual energy consumption needs.
Material innovations, such as developing hardware that requires less energy for data processing and storage and is easily recyclable, contribute to sustainability. These practices and innovations are essential in ensuring that AI growth does not come at an untenable environmental cost. AI data centers are becoming more environmentally friendly and sustainable.
Overall, the intersection of AI and data storage is characterized by rapid innovation aimed at meeting the technological, security, and sustainability needs of tomorrow. From quantum computing to DNA storage, and from edge computing to the integration of blockchain, these advancements are progressively reshaping the way AI data is stored and managed. It is within this evolving landscape that efficient and secure storage solutions for ai will play a pivotal role in enabling the vast capabilities AI promises, while adhering to essential ethical and ecological standards.